It’s no secret that organizations across the globe are undertaking wider and deeper enterprise migrations to the cloud. Cloud infrastructure services spending jumped 33% from US$107 billion in 2019 to US$142 billion in 2020, and 2021 shows no sign of slowing down. The reason for this growing demand can be summed up in a single phrase: real-time. Competing in today’s business world demands new levels of speed, agility, flexibility, and innovation – simultaneously – and the keys to unlocking those capabilities in real time are a modern cloud architecture with a cloud-first data analytics platform.
As cloud transformation continues to accelerate, in many cases transferring siloed data to separate, purpose-focused clouds, only a multi-cloud platform can equip enterprises to take maximum advantage of their future opportunities. A multi-cloud architecture effectively future-proofs organizations by enabling deployment flexibility and portability anywhere, including public clouds and on-premises servers, without locking an enterprise into a single cloud or architectural choice.
Building a data analytics platform that can scale across clouds is crucial to enabling any business user to answer any question at any time against any data. To achieve those goals, enterprises must modernize their cloud analytics architecture – a process with three key steps that are essential to success.
1) Modernize your cloud architecture
Several important factors work in unison to achieve a modernized cloud architecture: agility, self-service, and multidimensional scale. Let’s begin by looking at agility, which in this context refers to the ability to spin things up quickly in the cloud by creating an infrastructure, adding data to the infrastructure, then running ad hoc exploratory use cases using that infrastructure. All of this should be accomplished with no downtime, frictionless IT, a pay-for-what-you-use model, and the freedom to tear down test infrastructures and walk away without disrupting your primary, mission-critical data environments.
Second on the list of modernized cloud architecture features is self-service. Real-time enterprises now strive to democratize data and analytics across line-of-business users, developers, and data scientists. It’s fairly straightforward to offer multi-user interactivity with the system, including management of data sharing and collaboration, but more challenging to enable everyone to launch new workloads and run them at scale with frictionless support.
The third element of modern cloud architecture is workload management or multidimensional scale. Over the years, customer experiences have taught us that there is no such thing as “one-size-fits-all” when building solutions for real-time data processing. Some customers run operational analytics workloads that are more tactical in nature, requiring low latency and quick response times – serving these queries demands raw computing power and plenty of I/O bandwidth. Other customers run decision and support workloads that demand very high throughput and lots of memory, with different I/O and computing power needs.
For mixed workloads, dynamic resource utilization provides a means to better understand different workload needs and priorities, then gives them the right compute, memory, bandwidth, and latency resources to succeed. Memory allocation alone is critical in the cloud; fumbling this single factor can prevent some or many workloads from being processed in real-time. Multidimensional scalability delivers the advanced capabilities enterprises of the future need to run millions of productionized models on trillions of interactions every second of every day.
At the end of the day, a modern cloud architecture should offer agility with infinite elasticity, self-service capabilities, and the ability to keep workloads running smoothly at scale. So armed, companies can generate insights and answers that were previously impossible to achieve almost instantly.
2) Modernize your data management
Another step in the modern data analytics architecture journey is modernized data management. At its core, this is about breaking down data silos – providing access across a variety of data sources. Even when data is distributed, users need the ability to easily query real-time data across deployment models to augment their analytics.
Query federation simplifies access to data from multiple databases in the cloud and on-premises. Federation products separate the problem of access into a layer that can be managed independently of data storage and working areas, providing something akin to a “virtual hub” for data. The concept is to write one query that accesses two or more different databases to unify the results – to the user. It appears that one is accessing and joining tables from the same database. This capability is a differentiator compared with other cloud-native data warehouses because it helps support hybrid infrastructures where data is stored in many places.
Ideally, a data fabric will orchestrate on-demand data access at scale across multiple platforms in the analytics environment. Users can initiate queries from any linked platform and access any system it is connected to, combining data from multiple platforms in a single query, all without requiring that all the data be copied to one place first. This allows data to be accessed and transferred to and from participating systems, unlike the one-way data flow of data federation.
Using open data formats also makes collaboration easier; quickly and easily understanding and cataloging data sources and existing models before starting queries and new modeling speeds time to value; and ensuring that democratized and decentralized data – a key theme of modern data management – are secure and protected gives enterprises and their customers peace of mind that their data won’t end up in the wrong hands.
3) Modernize your analytics ‘“ at scale
Although data analytics fuel the enterprises of the future, much about the field can still be thwarted by the unknown. Data and data analytics functions can frequently happen in silos, making it difficult to operationalize. Consequently, cloud customers are constantly seeking ways to provide functions inside the platform and run them at scale to modernize their analytics and meet whole-enterprise needs.
For example, companies often face a significant challenge with data and analytics pipelines because developers and data scientists typically prefer cloud-native tools, or even their own tools, that may not be integrated with their primary platform. This preference has led to an emerging trend known as BYOA (Bring Your Own Analytics), giving cloud customers the flexibility to scale data across their enterprises.
Modern analytics also require better partner integration, which allows companies to integrate models more deeply, as well as exchange data between cloud-native services, analytics workbenches, and different analytics partners. Realistically, partners will help any enterprise complete the full scope of capabilities needed for real-time analytics. Companies should be on the lookout for platforms that not only connect with partners but also enable tight integrations with the platform to maximize performance by minimizing data movement and parallel computation.
Putting the three key modernization steps into practice
Enterprises have faced monumental changes over the past several years, and all signs point to continued change for the foreseeable future. Rapidly growing volumes of data from an increasing number of sources have presented both challenges and opportunities. Companies have emerged stronger after rising to the challenge by using scalable technologies to capture, manage, and optimize their data.
The enterprise of the future will leverage data as its most valuable asset, driving both real-time decision-making and innovations. But doing so will require approaching data analytics with a cloud-first mindset, leveraging a modern cloud data analytics platform that is scalable, and unifying all data in a means that is fully available for analytics.
A modern cloud architecture is fundamental to helping enterprises avoid future problems with lock-ins, like a single cloud solution or architectural choice that won’t seem as wise several years from now. One or more cloud providers will offer easy access to unlimited ‘” and independent ‘” compute and storage resources, deliver the capabilities and elasticity organizations need, and accomplish all of this with less management overhead. The result will be a faster, smarter, and more versatile data and analytics architecture that embraces cloud transformation, scaling smoothly as the trend and enterprise evolve over time.
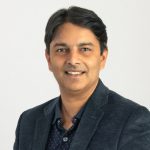