Sponsored by Instaclustr

Generative AI’s real-time capabilities will continue to redefine enterprise tech goals—particularly in analytics and data management. While AI/ML advances promise unprecedented automation and efficiency, it’s crucial to recognize this moment as the fourth major revolution in data analytics. Understanding past lessons can position leaders to better leverage generative AI and real-time data insights.
Bold leadership and adaptive cultures define the winners of data revolutions
At each turn in the history of data analytics, determined enterprise leadership and cultures capable of change have been as important to navigating modernization opportunities as the technology itself.
Historical context is worth understanding. Pre-1980s, enterprises were in the Data 1.0 era, making the initial shift from harnessing data manually to leveraging computer-powered automation. The focus of the organization was Automation the of manual processes. Companies making these first forays into the digital data world required leaders who understood the vision of computerized data and had the wherewithal to guide team cultures in embracing that future.
The arrival of formal processes turning data into business intelligence—alongside enterprise data warehouses and master data management—ushered in Data 2.0 from the 1980s until the 2000s. Organizations moved from just automation to gaining a Competitive Advantage through analytics capabilities, such that companies with leaders and cultures that lagged in this modernization were comparatively flying blind in the marketplace.
The mid-to-late 2000s was the beginning of the Data 3.0 era. The focus in this era was on Business Disruptions. Enterprises like Amazon, DoorDash, and Uber embraced the disruptive philosophy. It was here that data analytics revolutionized how data could shape customer experiences, drive operational efficiency, and even enable unprecedented business models. The success of disruptive new models also ushered in the modern dynamic of enterprise leaders viewing digital transformation as essential to building and maintaining competitive advantages. This era also made clear the stark contrast between the technology strategies and maneuvers required of established businesses versus those that were more nascent and nimbler. New organizations often start out utilizing the latest data analytics infrastructure, technologies, and techniques, while older enterprises must make decisive investments and cultural changes to turn their vast ships to a new course.
The current revolutionary push for generative-AI-powered data analytics—harnessing AI/ML and data science like never before—has ushered us into the Data 4.0 era. Businesses launching today can start with these tools and the powerful cloud infrastructures they require. For those that need to strategically modernize to adopt Data 4.0, the successful enterprises will be those that combine clear-eyed leadership and purposeful cultural change with the new technology, just as has proven true in every past cycle.
To harness Data 4.0 analytics, leaders must free data from Data 3.0 Constraints
At enterprises with Data 3.0 data analytics capabilities in place, data analysts must make requests from IT teams to access data. By the time IT teams follow governance procedures and finally enable analysts to utilize data stored in secure silos, the significant latency saddling that analysis often means opportunities are less ripe for the taking.
Data 4.0 offers data analysts the transformative power of self-service real-time data access—making it possible to quickly come to data-driven conclusions and take quick confident action. In this new era, technologies including self-service analytics applications, data replication and virtualization, cloud storage, and more offer analysts a buffet of powerful tools with which to adopt far more efficient practices. Data analysts also now have generative AI chat interfaces at their disposal, allowing them to ask conversational questions to quickly make sense of enterprise data.
Achieving the transition to Data 4.0 and tools offering direct data access demands that leaders embark on a technology and cultural transformation, introducing automation across the data supply chain that securely extracts, ingests, and prepares data that analysts can use immediately on-demand. That democratization of data access should reach personnel across organizations wherever real-time data analysis can provide benefits, and should be bolstered by automated operations and data security measures capable of spanning even multi-cloud infrastructure.
Even with Data 4.0’s clear competitive advantages, enterprise silos are famously difficult to demolish—and established team culture can be just as hard to uproot. With legacy Data 3.0 practices where an analyst must send another human a request for data and then wait, replacing that system will require trust and buy-in from both the analysts and those security-focused gatekeepers. Leaders who champion and instill goals based not on processes but on end results will have the easiest time in completing their Data 4.0 transitions.
Forecasting the impact of maturing AI/ML capabilities out into the near future, Data 4.0 implementations will likely automate data pipeline management in just a few years. With AI/ML handling everything from initial data capture to analytics and preparing insights, data analysts will be able to focus on dissecting real-time data to improve customer experiences and internal operations. Getting this new optimization and efficiency correct will enable businesses to run circles around competitors stuck with Data 3.0 tools and processes.
Prove the technology to move the culture forward
Across every past data revolution, leaders who take the time to prepare their internal cultures for oncoming technology shifts have had the greatest success. Establishing the human infrastructure—building buy-in across teams and stakeholders by proving the value of the technology and sharing a positive vision of more intuitive and fluid workflows and practices—is as essential as any technological infrastructure. On the other hand, leaders that fail to do the groundwork and nevertheless press new technologies and practices upon company cultures that aren’t ready to accept them are in for a rougher ride.
This is as true with pursuing AI/ML and generative AI goals as it was with first adopting big data, or the first BI tools, or computers themselves. And, once again, the competitive edge those effective leaders achieve will be unlike anything that’s existed before.
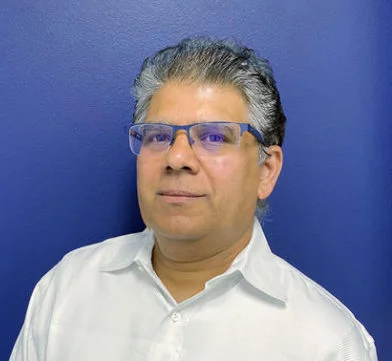
Anil Inamdar is the Head of Consulting Services at Instaclustr by NetApp. Anil has 20+ years of experience in data and analytics roles. Joining Instaclustr in 2019, he works with organizations to drive successful data-centric digital transformations via the right cultural, operational, architectural, and technological roadmaps. Before Instaclustr, he held data & analytics leadership roles at Dell EMC, Accenture, and Visa. Anil lives and works in the Bay Area.