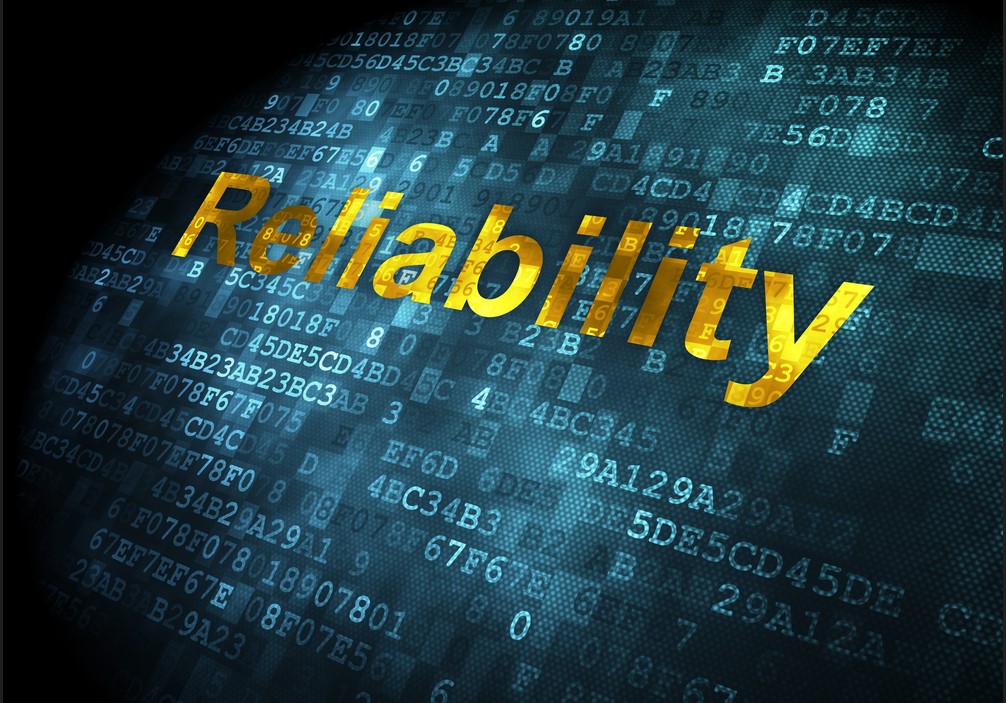
While companies are transforming their workloads to hybrid and multi-cloud environments, they still treat operations as silos between infrastructure, applications, and data. To manage the complexity with a resilient, reliable, secure, and cost-optimized approach, organizations need all three to go hand in hand.
Unfortunately, before that can happen, each of the areas must get equal treatment. And that has not been the case with data. Businesses have invested great amounts of time and money in planning, developing, testing, and deploying infrastructure and apps. But not as much attention has been paid to the data aspects of their operations.
Learn more about Hitachi Application Reliability Centers (HARC)
Why is this so important? There are multiple reasons.
Many businesses today aim to be data-driven. They make strategic decisions by analyzing the vast amounts of data available from numerous sources such as smart sensors, IoT devices, social networks, website clickstreams, customer interactions, and more. The sources and quantities of data are increasing as a result of the wide-scale embracement of digital transformation.
Inaccurate data and its lack of availability to needed data can impact business success or failure. A bank calculating a suitable rate for a loan applicant could lose a good customer or lock in a risky one if the data the analysis was based on is outdated or inaccurate.
Another factor that impacts data reliability is the complexity of modern applications. Cloud-based apps and workloads are often composed of modular elements and distributed systems and often make use of multiple data sources.
The complexity makes it hard to see the relationship between data and outcomes. An interesting example from the pandemic illustrates the point. Medium and short-term computer weather models started having unusual inaccuracies during the pandemic. It turns out model accuracy was aided by wind direction and speed, air pressure, temperature, and humidity measurements collected globally by commercial airlines and cargo planes. This was not obvious due to the expansiveness of the application and the data sources used as input. It took an extensive investigation to figure out what was happening.
Most businesses do not have the resources of government agencies to track down such data issues. Yet, their hybrid and multi-cloud applications and distributed data sources are just as complex.
These are areas where data reliability engineering can help.
The emergence of data reliability engineering
Historically, data issues were relegated to data engineers, data scientists, and analytics experts. These groups did not have the tools and processes at their disposal that other teams like SREs or DevOps already made use of in their respective infrastructure and application arenas.
Learn more about Hitachi Application Reliability Centers (HARC)
Thus emerged the need for a functional entity called data reliability engineering. Borrowing from SRE and DevOps and bringing data into the fold just as these groups did with infrastructure and applications, data reliability engineering is the practice of delivering high data availability and quality throughout the entire data life cycle from ingestion to end products.
A data reliability engineer (DRE) looks for errors in a company’s data operations, seeks to ensure data reliability and quality, and makes sure data pipelines are delivering fresh and high-quality data to the users and applications.
Additionally, by adopting Data Reliability Engineering (DRE) best practices, DREs can show the internal stakeholders data’s importance to the organization. And as is the case with SREs and DevOps, a DRE team should develop KPIs and metrics data for data availability, data completeness, and data downtime.
And also, just like SRE and DevOps, DREs must use a variety of tools and methodologies to be successful. For example, data observability tools can help provide visibility, identify data problems, optimize data usage and capacity planning, and help achieve data trust.
Additionally, there is a need for tools to automate data policies to guarantee data availability, reliability, and quality. Automation is also needed to identify root cause issues, self-correct problems, and self-heal data flaws to enable enterprises to move faster and reliably.
See also: Data Engineers Spend Two Days Per Week Fixing Bad Data
The need for a technology partner
DRE is a critical but emerging field. It requires a variety of skills and tools and greatly benefits from proven best practices and topic area knowledge.
Unfortunately, many businesses find they do not have the internal expertise or resources to undertake DRE efforts. And as such, they seek the help of a partner.
Learn more about Hitachi Application Reliability Centers (HARC)
Increasingly, help is coming from organizations and providers that provide an integrated portfolio of cloud and application professional and managed services offerings designed to help businesses address the complexity of modern cloud infrastructure, application, and data environments.
Ideally, the partner needs a wealth of experience that is complemented by best practices shared with their clients. In some cases, a partner might have a center of excellence where accumulated knowledge and expertise are turned into well-honed playbooks, best practices, implementation guides, and more.
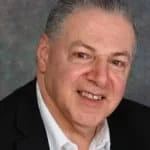
Salvatore Salamone is a physicist by training who has been writing about science and information technology for more than 30 years. During that time, he has been a senior or executive editor at many industry-leading publications including High Technology, Network World, Byte Magazine, Data Communications, LAN Times, InternetWeek, Bio-IT World, and Lightwave, The Journal of Fiber Optics. He also is the author of three business technology books.