Businesses are collecting more data than ever before, with IDC and Seagate projecting a 42.2% annual growth rate in enterprise data collection over the next four years. However, without a proper framework in place, a lot of this data ends up going unused or fails to reach the right destination. At a time when organizations are moving data to the cloud and experimenting with artificial intelligence and machine learning, the need for component data architecture processes is more critical than ever before.
What is data architecture?
Data architecture is the framework organizations use to document all data assets and govern how these assets are stored, arranged, and integrated into other data systems. The implementation of standards, rules, and policies on data assets that flow into an organization can provide a clearer view of what is happening inside the business, enabling faster analysis and visualization of data, and permit all teams to make data-driven decisions without the need for a complete understanding of the data process.
Modern data architecture practices are built for applications that require real-time processing and visualization by integrating various data pipelines into a unified platform that can be accessed by all those in the company. The use of container orchestration tools automates much of the operational effort to run workloads and services, and data fabric or mesh technologies are the newest data-as-a-platform layer that fills the gaps in unifying an organization’s data flows.
Why is data architecture important?
Without data architecture, businesses often end up collecting a huge amount of unstructured data, which fails to achieve the goals set out by the company. This happens all too often, as organizations regularly take a ‘technology-first approach’ to data collection, according to McKinsey, and rush to implement new digital technologies without going through the necessary processes of designing data architecture and implementing infrastructure to support data and analytics at scale.
This can lead to all sorts of issues further down the pipeline, including redundant and inconsistent data storage, in which an organization lacks a single source of truth and has similar datasets stored in numerous data silos. This lack of focus can also lead to development sprawl, where a single function requires multiple technologies to support it. As a business gets larger, these issues will be magnified and can lead to lower productivity, as more technologies inevitably leads to more potential blockages in the data pipelines, and new employees need to be taught how to use more technologies.
What are the benefits of using modern data architecture?
Modern data architecture has many benefits to data leaders looking to implement the new wave of collection, analytics, and visualization technologies into their businesses.
- Automation – The amount of data that digital organizations can collect today is far more than any manual process can handle, and many automation tools have been built with modern data architecture practices in mind to handle the workload.
- Unified dashboard – All stakeholders should be able to access data regardless of the platform or system, improving collaboration and real-time access.
- Performance and productivity – Modern data architecture enables the handling of data-intensive workloads, such as AI, ML, and data analytics platforms.
- Scalability – Modern data architecture allows businesses to scale to meet current demands, while the infrastructure does not need to be tied to specific platforms or environments as it was in the previous generation of data collection.
A look to the future
One of the key reasons business leaders bring up for failing to reach their businesses analytics goals is lack of data architecture. This lack of focus on the data strategy from the start is likely going to cost businesses even more than it did a decade ago because of the cost of storing more data than ever before and running it through analytics platforms. The creation of modern data architecture practices, such as data fabrics and data meshes, has provided smart organizations with the power to collect data from disparate sources and process and display it in one unified platform.
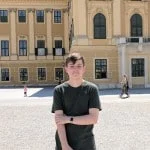