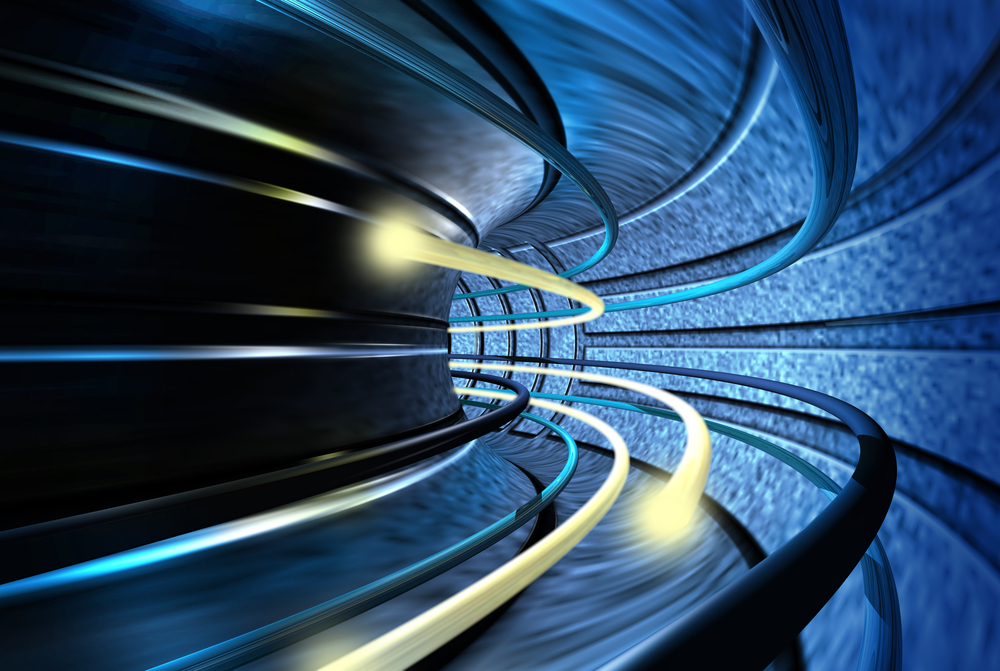
How we create, manage, and leverage pipelines is undergoing profound transformation. The interplay between cutting-edge technology like artificial intelligence and evolving business needs has triggered a series of trends reshaping how organizations approach the entire concept of data pipelines. It’s not a choice. Companies must abandon old ways of doing things to continue to make the most of big data and operate competitively. One of the most egregious right now is the overreliance on manual creation of data pipelines. Here are the key reasons why this traditional approach must make way for the new era of automated, AI-driven solutions.
Modern pipelines are shifting towards automation, driven by AI
What’s the priority with today’s data pipelines? Most likely, you answered “successful scale and management of complexity.” Automation is the key ingredient that makes this happen, and when that automation is supported by AI, great things can happen. Organizations need near-instant decision-making capabilities without sacrificing data quality. This is pushing the boundaries of data pipelines.
Traditional manual development, often reliant on batch processing, struggles to keep up because of:
AI-driven processes and automation are making manual creation a thing of the past. AI handles proactive monitoring, enables accurate anomaly detection for safer data handling, and includes self-healing processes, all of which reduce the need for continuous human oversight. They don’t replace humans completely, but they help carry the load and manage complexity so humans can do what they do best—problem-solve and make decisions.
See also: Challenges Data Pipeline Engineers Need to Overcome
The emphasis on real-time processing and data quality demands a different approach
Real-time processing and data quality are two critical pillars of the modern pipeline process. Organizations need instantaneous insights for quick decision-making, which means they need pipelines that can handle real-time data processing and analysis. Manual pipeline creation is rooted in processes that are inherently slower, less agile, and prone to human errors. Digital transformation requires precision, speed, and responsiveness only achievable through automation and AI-driven solutions. Manual development will fall short because:
Working with data requires holistic data governance plus agility
Modern data pipelines go beyond just data processing. They’re rooted in holistic data governance and agility. Companies rely on standardized practices, collaboration, and compliance through well-defined data governance frameworks — trusting data handling allows them to treat data as a dynamic asset rather than a static liability.
Data quality and standardization
Manual development: Humans are good at many things, but consistency isn’t always one. Data needs change. Data sources change. Even development teams change. Manual processes often lack consistent data quality checks, leading to errors.
AI and automation: AI algorithms can identify and correct data quality issues in real time, ensuring standardized and (most importantly) reliable data flows. Automated data profiling and cleansing processes maintain high data quality throughout the pipeline. With this support in place, humans can take on higher-level quality checks and monitor for bias in results.
Real-time monitoring and anomaly detection
Manual development: Human developers are tied to monitoring data streams, a time-consuming activity that sidelines higher-level tasks and is prone to errors.
AI and automation: AI-driven monitoring tools continuously analyze data streams in the background, detecting anomalies and deviations promptly, no matter how large the ecosystem is. Automated alerts and responses ensure developers take rapid, corrective actions to maintain data integrity and prevent cybersecurity incidents.
Adaptive integration and resource optimization
Manual development: Human teams may struggle with resource optimization in today’s varying workloads and complex data ecosystems. There’s just no way to adapt in real time to evolving data sources, formats, requirements, tools, and needs.
AI and automation: AI can adjust processing logic and accommodate changes in real time. These algorithms learn from data patterns and get more efficient over time. Additionally, these platforms can allocate resources based on demand to prevent performance bottlenecks during peak times.
Regulatory compliance and privacy
Manual development: Once again, humans can’t always keep up with changing regulatory and compliance demands, at scale, with the current complexity of the data ecosystem.
AI and automation: AI-driven processes enforce compliance by automating best practices like data masking, encryption, and access controls. This reduces the risk of breaches but makes data available to required stakeholders for decision-making.
Precision, speed, and adaptability will win
AI and automation are transforming data pipeline creation. Manual development is becoming a relic of the past, with slow processing, data inconsistencies, and lack of agility preventing companies from harnessing digital transformation. Organizations that embrace the shift away from manual pipeline creation will position themselves to harness the full potential of data assets and stay ahead in the data-driven journey that will define the future of operations.
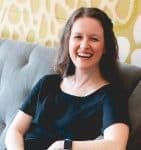
Elizabeth Wallace is a Nashville-based freelance writer with a soft spot for data science and AI and a background in linguistics. She spent 13 years teaching language in higher ed and now helps startups and other organizations explain – clearly – what it is they do.